Building the next decade of consumer finance
I had the great pleasure of moderating a panel at Fintech Meetup re: building the next decade of consumer finance by leveraging real-time data and cash flow forecasting. I wanted to share some of the key insights shared during the session by our amazing panelists...
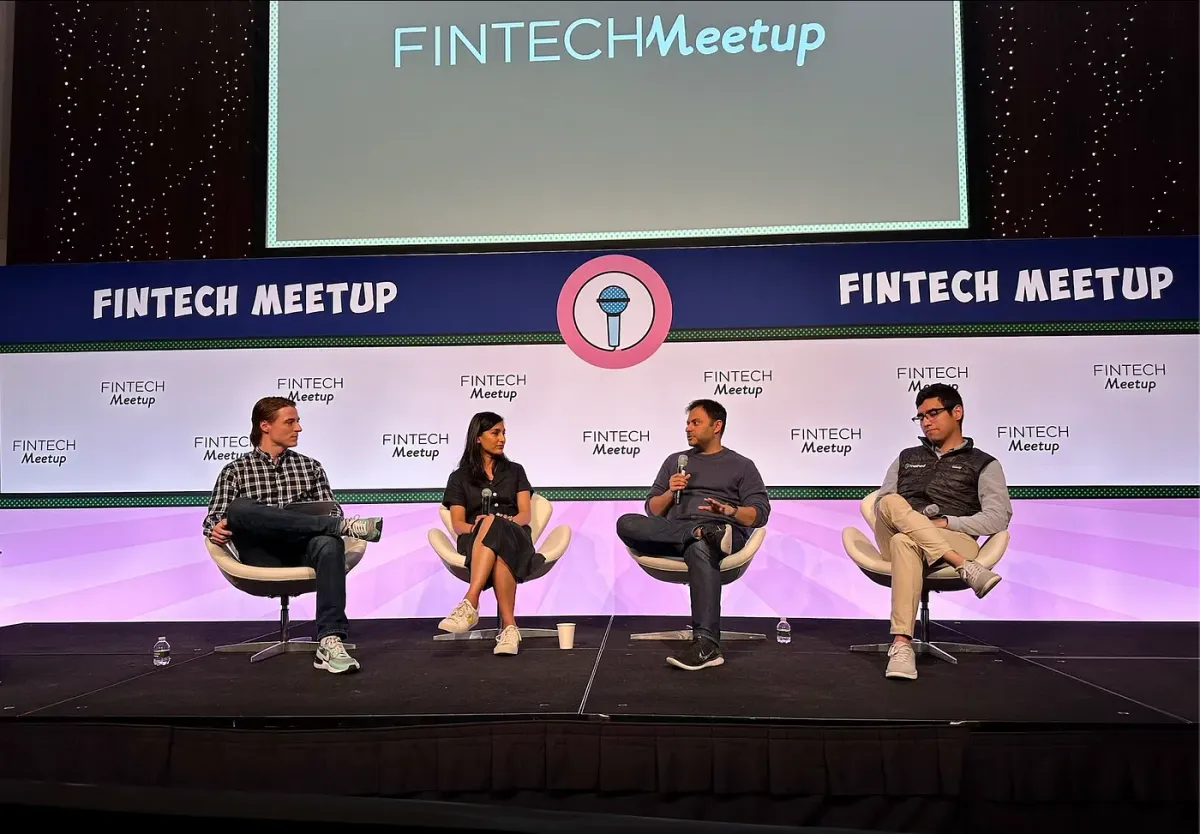
Last week in Las Vegas, I had the great pleasure of moderating a panel at Fintech Meetup re: building the next decade of consumer finance by leveraging real-time data and cash flow forecasting. I wanted to share some of the key insights shared during the session by our amazing panelists, Jose Bethancourt (Co-Founder of Method Financial), Ema Rouf (Co-Founder of Pave.dev), and Zane Salim (Co-Founder of Atlas)!
1/ Alternative data augments FICO across the entire credit spectrum — this is about FICO+ NOT replacing FICO.
The issue of credit invisibility in the U.S. is a well known and documented problem and it is only getting worse. Many fintech companies talk about acquiring users earlier in their financial journeys, but can’t actually underwrite them because these consumers have never engaged with credit products and therefore are “no file” or “thin file.”
Experian and Oliver Wyman estimated that 28 million adult Americans are credit invisible and 21 million are unscorable. So, we need alternative data, which in this context just means non credit-bureau data (e.g., income, employment, bank account, etc.), to make decisions about these consumers and offer them financial services and products. Companies have already popped up to solve parts of this problem (e.g., Nova Credit which translates credit history from other countries for immigrants).
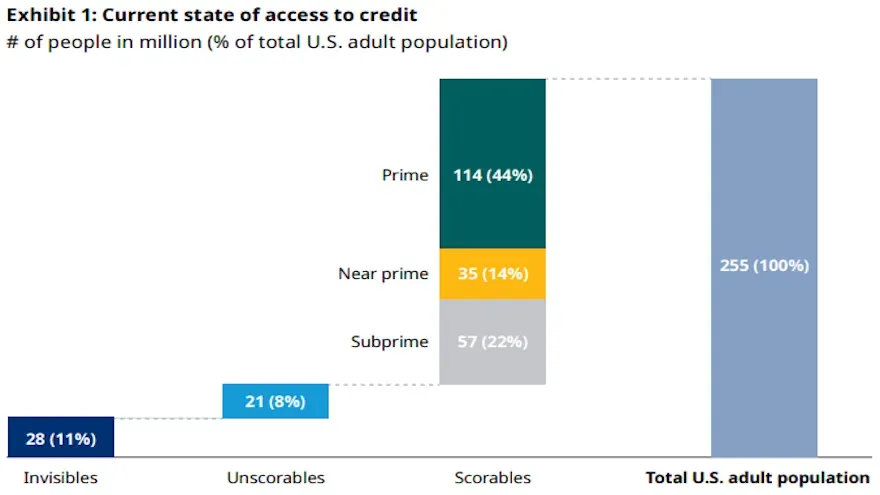
However, alternative data can be used for other parts of the FICO spectrum as well, not just the credit invisibles. Anecdotally, many folks in the risk space have identified and already seen the impact of degradation of credit bureau data and are positing that borrowers with even low prime scores aren’t as creditworthy as they once were.
In the current period of economic stress, lenders will tighten credit approvals to lower delinquencies and credit losses. And demand for credit products is likely higher — negative income shocks often result in drawing on new or existing credit to smooth things out. So, lenders who want to continue to grow, sustainably and responsibly, need to make better risk-weighted decisions for many segments of users, not just credit invisible ones, and alternative data offers an opportunity to do exactly that.
2/Real-time data powers better products and outcomes by enabling greater access and improving the quality of risk management.
Folks in this space know that bureau data can lag pretty substantially — 45 days (or more) depending on what bureau report or product is being accessed and the furnishing timelines of the lenders. So, pulling a credit report doesn’t give a lender insight into the most recent behavior of a borrower. What if, within the last 45 days, the borrower had demonstrated positive repayment behavior (e.g., repaid several outstanding debts)?
As an example, Atlas, a payroll powered credit card, accesses real time data, including cash flow and payroll data, on consumer financial health to continuously monitor their users. Doing so can help the company discern if a users’ financial position has changed and, if needed, change their credit limits. This ability to access real time data allows for better risk management and can even serve to prevent losses before they happen.
3/ The movement to make alternative data mainstream has to happen outside of the credit bureaus.
This isn’t to say that the bureaus will disappear or be disintermediated! However, there is a lot of data that isn’t actually furnished to the credit bureaus, so credit reports just don’t provide a comprehensive view of a consumer’s debt obligations. For example, most BNPL loans aren’t currently being furnished to the bureaus. And, each of the bureaus has published some sort of announcement regarding their approach to integrating BNPL data (Equifax, TransUnion, Experian), so who knows what standard, if any, will be adopted, and how long it’ll take to get there (for BNPL data and other types of alternative data).
As an example, Method collects consumers’ liabilities across over 60k institutions so that lenders can access all of a person’s debt obligations by combining data from credit bureaus with financial institutions’ core banking systems. So, if a consumer had recently taken out several loans, a lender would know, whereas that data might not show up for 45 days, or more, in a credit report, helping to prevent loan stacking.
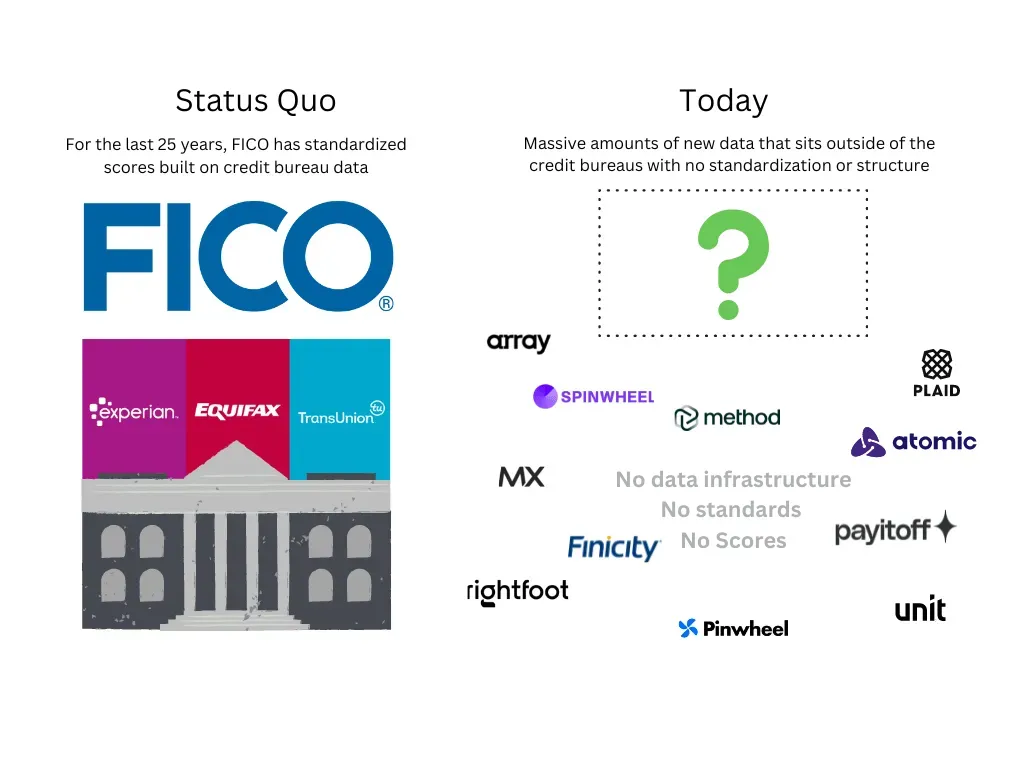
4/ Recent innovation in infrastructure has made this data far more accessible than it has been in the past.
Many fintech companies experimented with using bank transaction data from Plaid, Yodlee, etc. years ago and ended up walking away from the data. Generally, the takeaway was that the data was incredibly messy, people didn’t know how to use it, and no one wanted to dedicate engineering resources to standardize the data. However, the infrastructure and tools available to access and enrich this data and make it easier to use have progressed substantially in the last few years. Admittedly, there is still infrastructure that needs to be built to help fintechs and banks adopt and use this data in decisioning (if you’re building in this space, let’s talk).
As an example, both Method and Pave are providing the infrastructure that has made it easier for companies, like Atlas, to access, interpret, and use this data. While Method collects and standardizes debt obligation data, Pave offers transaction enrichment which serves to ingest a raw transaction and return clean transaction data. These infrastructure providers, and others, that allow for real-time access to data used in decisioning, such as Monnaiand Effectiv, are serving to dramatically accelerate adoption.
5/ Mature lenders don’t want scores, they want raw data or attributes.
While some fintech companies that are just getting started are willing to make underwriting decisions based on scores so that they can get a product to market as quickly as possible, most either want the raw data, so that they can use it to develop new or enhance their own models, or the underlying attributes that inform the scores. If they can’t understand the scores, then they can’t explain them, which presents challenges when trying to get originating banks or capital providers to buy in. Furthermore, attribute generation on top of clean transaction data and unifying transactions with other sources like liabilities and credit report attributes abstracts more levels of data engineering and data science to help a team move faster on model development.
As an example, Pave offers transaction cleaning and enrichment and also recently launched their own attributes toolbox — lenders can use their Attributes Store in their proprietary models. This level of flexibility in terms of how companies can use and understand the data similarly will serve to accelerate adoption.
In conclusion, the use of real-time data and cash flow forecasting is becoming increasingly important in consumer finance. Alternative data, infrastructure innovation, and a focus on the user experience are key to making these advancements work for both consumers and lenders.